Volume 1, Issue 1
Navigating the Integration of Artificial Intelligence and Surgery
By Ishan Patel
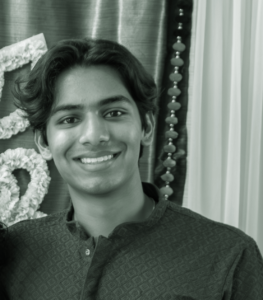
is a fourth-year student majoring in Management Information Systems. Their research area encapsulates biology and microbiology work, particularly within the healthcare field. Their work is advised by Dr. Mindy Adnot from the Honors College, Dr. Srijan Das from the College of Computing and Informatics, and Professor Andrew Goff from the Department of Biological Sciences.
Abstract
Integrating artificial intelligence (AI) into surgery presents a promising avenue for enhancing diagnostic and therapeutic practices, yet challenges persist in advancing this technology in a surgical setting. Despite strides made in AI-based platforms for medical imaging, robot-assisted laparoscopic surgery, and anesthetic calculations, AI for surgery remains elusive due to limitations in existing optimization approaches and potential ethical issues that arise from utilizing such tools on patients. This literature review investigates the feasibility of utilizing AI to optimize surgery, particularly in aligning 3D images, assisting surgeons with breakthrough techniques, and highlighting certain areas of the body that surgeons have a hard time seeing while operating at the same time. Preliminary research reveals that leveraging an AI agent matches, and sometimes outperforms, current state-of-the-art methods in accuracy and robustness for aligning images in patient screenings and diagnoses. These findings highlight the potential of AI-driven image registration techniques to revolutionize medical imaging, offering insights into addressing long-standing challenges and paving the way for more effective utilization of AI in surgical settings.
Additionally, it highlights the importance of continuous ethical considerations in the deployment of AI technologies in patient care, emphasizing the need for ongoing revisions to ethical codes to accommodate the evolving landscape of machine learning systems and technology. This review contributes to understanding the transformative impact of AI in a surgical environment while emphasizing the importance of ethical frameworks in harnessing its full potential.
Artificial Intelligence, Surgery, Ethics, Laparoscopic Surgery, Anesthetics, Remote Patient Monitoring
Introduction
In recent years, the intersection of artificial intelligence (AI) and surgery has garnered significant attention and intrigue. The surge in AI applications across various sectors, coupled with the pressing demand for advancements in surgical practice, highlights the importance of exploring this emerging field. As AI technologies continue to evolve, their potential to revolutionize surgical decision-making, patient care, and operative techniques becomes increasingly evident (Mirnezami et al., 2018; Zhou et al., 2020).
Amidst this backdrop, a critical unresolved question emerges: How can AI be effectively integrated into the overwhelmed healthcare system to augment patient care through surgical procedures? How can it also navigate the ethical concerns that arise from autonomous patient care? The integration of AI into surgical workflows presents both opportunities and complexities (Mirnezami et al., 2018). While AI holds the promise of revolutionizing surgical decision-making, enhancing precision, and streamlining operative techniques, it also raises ethical dilemmas, regulatory hurdles, and concerns regarding human-AI collaboration (Rudzicz & Saqur, 2020). This inquiry goes beyond assessing the technological feasibility of AI in surgery. It also examines its impact on patient safety, healthcare delivery, and the evolving roles of surgeons and autonomous robotic systems.
Evidence from existing literature highlights the multifaceted applications of AI. Disciplinary fields such as computer science and medicine have explored the benefits and challenges of AI in surgery. While some research has been conducted on AI applications in healthcare, including medical imaging and diagnostic support, the specific inquiry into its integration within surgical practice remains relatively less studied (Liao et al., 2016). Existing literature offers insights into AI-driven advancements in surgical robotics in laparoscopic surgery (Biebl et al., 2021), anesthetic calculations (Dominikowski et al., 2021), patient monitoring (Shaik et al., 2023), and medical image analysis and overlap (Liao et al, 2016), highlighting the extensive applications of this technology.
While there is a small amount of research currently, the potential remains that AI will have a large impact on the healthcare industry. Most of these research studies have multiple follow-up questions that are important to answer in the future but show that AI can lead to a large breakthrough in bettering patient care. The main hurdle that most research studies encounter in this field is that patient testing is very limited due to certain ethical restrictions. Thus, the process of incorporating AI into surgery will be a slow transition before more techniques are introduced and researched (Mirnezami et al., 2018; Rudzicz et al., 2020).
This literature review attempts to explore the following aspects of AI’s surgical revolution:
- Considerations and challenges with AI’s integration into healthcare
- AI’s potential uses for laparoscopic surgeries
- Saving time in the operating room and emergency department
- Automating anesthetic calculations and patient monitoring
To examine these questions, this literature review draws upon interdisciplinary sources from fields such as computer science, biomedical engineering, and surgical medicine. Given the rapid advancements in AI, newer research is particularly relevant.
Therefore, most sources were selected from within the past five years, with some foundational or conceptual studies included from the last ten years. Peer-reviewed journals were prioritized, with articles sourced through the J. Murrey Atkins Library and databases such as PubMed and Web of Science. Additionally, relevant sources were identified by reviewing the reference sections of key articles, allowing for the discovery of studies that addressed specific aspects of AI’s role in surgery. Selected studies were evaluated based on their credibility, empirical rigor, and contributions to ongoing discussions on AI in surgical applications. Preference was given to studies providing data, systematic reviews, and emerging trends to ensure a well-rounded investigation of AI’s impact on surgical practice.
To provide a comprehensive analysis, this review is structured around four key areas where AI has significant potential to transform surgical practice, selected based on both practical implications and existing concerns surrounding AI integration. Ethical considerations were included because AI remains a controversial topic in many fields, especially in medicine. Addressing issues such as transparency, bias, and human-AI collaboration is essential for widespread adoption. A focus on laparoscopic surgery was chosen due to its reliance on cameras and other visual technologies, which present opportunities for AI-driven enhancements in visualization, surgical guidance, and automation. Time efficiency in the operating room and emergency departments was selected to explore AI’s potential to streamline procedures and reduce stress on hospital systems and surgeons. These are critical factors in improving patient outcomes. Lastly, AI’s role in anesthetic calculations and patient monitoring was examined because anesthesia involves complex dosage calculations, and AI’s predictive capabilities may enhance both intraoperative and postoperative care. Within each subsection, studies were selected based on their contributions, ensuring that the review presents both theoretical insights and data-driven evaluations of AI’s role in surgical advancements. This literature review aims to enhance understanding of AI’s transformative potential in shaping the future of surgical care and patient treatment, while highlighting considerations that need to be taken before AI is fully integrated into healthcare.
Key Considerations and Challenges with AI’s Integration into Healthcare
Prior to the complete integration of AI in healthcare, it is important to remember that there are many shortcomings in AI algorithms and ethical considerations that need to be addressed. The first challenge is that many studies that are currently utilizing AI have been published on preprint servers without being submitted to peer-reviewed journals. These studies often lack randomized controlled trials (RCTs) of AI systems but rather use small, prospective studies. Randomized controlled trials minimize bias and provide a strong correlation between interventions and outcomes. Medical professionals mainly trust peer-reviewed journals with RCT, so in the future, more trials need to be conducted using this method (Kelly et al., 2019).
Another challenge that exists with AI is the potential for algorithmic bias. AI systems are trained on sets of data, but if these data sets are limited to potential groups of people, patient diagnosis could be affected. In the healthcare field, many groups are already disadvantaged by factors such as race, gender, and socioeconomic background. If AI algorithms are trained off of biased data, it makes it harder to diagnose these patients on an equal level compared to patients who have more data for their background. One study found that hospital mortality prediction algorithms have varying levels of accuracy based on ethnicity (Kelly et al., 2019). AI algorithms also tend to correlate unrelated information to their diagnosis. Another study showed that an algorithm had a higher chance of falsely classifying a skin lesion as malignant if the image had a ruler in it. This was because the model was trained on images where many cancerous lesion pictures also contained a ruler within the image (Kelly et al., 2019). These discrepancies within AI algorithms need to be addressed before they can be fully utilized by doctors and surgeons for patient care.
Patient medical records and medical scans are sensitive information that warrants careful handling. If this information is stored in an AI-based algorithm, there can be potential data breaches that can leak patient information (Rudzicz et al., 2020). Many attempts have been made to de-identify patients from their data. The problem persists that algorithms can re-identify individuals and match their data with their records from as little as 3 data points (Crigger et al., 2019). Medical scans can even be altered in malicious ways which can alter the algorithms of AI systems. An AI algorithm misdiagnosed an image of benign moles as malignant when the picture was rotated (Kelly et al., 2019). Something as simple as photo alterations could create trouble in patient care when a diagnosis is simply based on these algorithms alone.
It is important to find the balance between maintaining human oversight as well as creating ethical rules in place for this evolving landscape. Before this technology is deployed into patient care, AI needs to be able to promote the quality of care without creating disruptions. When AI models make predictions, it is also important to explain how those algorithms came to that conclusion to allow for more trust between physicians and AI. This technology should be seen as an additional tool to treat patients rather than a replacement for doctors, so this level of comfort should be sought after. Although many parts of AI may cause concern, many promising innovations will help surgeons treat their patients.
Using AI in Laparoscopic Surgeries
AI is gradually gathering more information on human anatomies and how surgical techniques are completed, with its capabilities expanding beyond current models. As more and more information is added into AI algorithms, the newer AI models will have more accurate information and data sets. With the ability to assist in surgical decision-making, one part of the body has been getting tested more frequently using AI decision support systems. For the gastrointestinal (GI) system practices, personalized treatment decisions are being generated through AI. One such example of personalized treatment involves artificial intelligence analyzing a patient’s genetic sequence to figure out drug and gene-specific interactions. These relationships are utilized to minimize the side effects of pharmaceutical drugs. Generated decisions are then paired with the knowledge that surgeons already have to allow for the best decision-making (Mirnezami et al., 2018). The newest method of GI surgery is laparoscopic surgery, or surgery done using small incisions while viewing inside the body using a camera. Laparoscopic surgery as a whole is important, as it is minimally invasive, reducing blood loss during operations and pain post-procedure (Biebl et al., 2021). This reduction in complications and pain allows patients to recover more quickly while requiring fewer medications.
Before AI can be used in surgery, it has to be trained on the material it is learning using video analysis. Surgeons use laparoscopic videos of surgeries for training, but the process is hindered by the need for a human to review the footage and manually create educational annotations. This process is time-consuming, so machine-based AI can be used to streamline this process by analyzing the videos (Mirnezami et al., 2018). Videos of laparoscopic colorectal surgery (LCRS) were used to evaluate the accuracy of automatic recognition of the body using AI. In this research trial, 300 surgical videos were used, coming out to over 82 million frames being analyzed and annotated by AI. 80% of the frames were being used for the training dataset and the other 20% were used as a testing dataset. To analyze the frames, researchers used a convolution neural network (CNN), which is an AI model. The overall accuracy of this automated surgical analysis was 81%, which left some room for improvement but demonstrated an optimistic outlook for streamlining this process (Kitaguchi et al., 2020). One potential drawback that was mentioned earlier is algorithmic bias. This complication can potentially occur within this AI model that would negatively affect the accuracy of the model.
In order to apply this information to another project, a study was completed where an AI model called Eureka was developed to separate nerves in the GI system visually. The AI model used the same training videos that surgeons use as an educational tool for laparoscopic colorectal surgery (Ryu et al., 2024). This training model was used in colorectal cancer patient videos, where the nerves of patients are important anatomical landmarks that help surgeons see where to dissect. If a surgeon was untrained and caused nerve damage to this area by accident, it could lead to catastrophic issues such as urinary and/or sexual dysfunction (Ryu et al., 2024). After the results from the study were collected, it showed that the total mesorectal excision (TME) was safely performed by the surgical trainees with the help of AI navigation, with no postoperative complications occurring. When the trainees couldn’t recognize the hypogastric nerves or the lumbar splanchnic nerves, the AI model aided in anatomical recognition in all cases. However, in recognizing the pelvic visceral nerves, the AI model only aided the trainees 25% of the time (Ryu et al., 2023). This research study helps to highlight the importance of AI in assisting in minimally invasive procedures, as there is less risk to patients and it has a high ceiling for improvement.
Using AI to Save Time During Operations and in the Emergency Department
Currently, during operations, surgeons require complex hand-eye coordination skills to look away from the surgical field to verify the position of the navigated instrument position on a monitor (Siemionow et al., 2020). This causes a high learning curve to be established for new surgeons, which removes time from actually learning and performing the operation. The movement of looking back and forth between a monitor and the patient also increases the amount of time in the operating room. A cross-sectional analysis was completed to see how much it costs a California hospital for operating room time. The results from the 2014 study showed that the average cost of OR time is $36 to $37 per minute, with around $20 being direct costs, $13 to $14 attributing to wages and benefits, and the other $3 coming from surgical supplies (Chu et al., 2022). By removing this unnecessary complexity of constantly looking at a monitor during the procedure, the duration of the operation will decrease. This saves money in multiple aspects, including anesthetic costs, and will eventually lead to lower medical bills for patients (Chu et al., 2022).
One way to fix this issue is by utilizing medical image registration, where two or more images are aligned. A research trial was created in 2016 that utilized an abdominal CT scan and a cardiac CT scan, and the goal was to see if AI could outperform human performance when aligning the medical images onto the patients. In both examples, the artificial intelligence agent outperformed current methods by a large margin, both in accuracy and robustness (Liao et al., 2016). Augmented reality is the new way to align medical scans. An augmented reality and artificial intelligence (ARAI)-assisted surgical navigation system was tested in a research trial to assess its accuracy and feasibility (Siemionow et al., 2020). ARAI creates a display system that hovers over a surgical field, projecting 3D medical images that correspond with the patient’s anatomy. ARAI’s navigation probes were tested in 4 cadaveric spines, and the probes were correctly placed in all trials (Siemionow et al., 2020). This shows how the augmented reality virtual overlay accurately corresponded to the 3D-generated image using AI in all tests.
Emergency departments are also areas where managing patient care using AI can shorten wait times. In most cases, patients wait for longer periods, while the actual diagnosis and treatment take a much shorter amount of time (Li et al., 2021). A study was conducted in China in which a natural language processing model (NLP) was created based on deep learning to extract clinical information from electronic medical records. Then, an AI algorithm was built based on the extracted information, which was called XIAO YI. This algorithm could take the information given by patients and determine the corresponding examination or test items that were needed based on that patient’s current health problem. In this study, waiting time was defined as the time from registration to preparation for the imaging examination or lab test. Utilizing XIAO YI led to a reduction in patient wait times from 2 hours to 0.38 hours at Shanghai Children’s Medical Center (Li et al., 2021). Ultimately, this allows for more patients to cycle through the emergency department, creating space for patients with more life-threatening issues.
Automating Anesthetic Dosage Calculations and Patient Monitoring
Another way to reduce time spent in the operating room is by automating anesthetic dosing, which streamlines a process that would otherwise require continuous manual adjustments by anesthesiologists, potentially saving crucial minutes during surgery. An experiment was completed that automated anesthetic inhalation for patients in order to reduce human errors. Some other benefits of automating this process include increased safety for patients, reduced costs, and less environmental pollution that is released from the process. This procedure was done under the supervision of an anesthesiologist during a laparoscopic operation, where the release of desflurane (anesthetic gas) could be controlled automatically. Currently, anesthesia machines have a monitor to analyze amounts of inhaled anesthetic gas and oxygen during inhalation and exhalation (Dominikowski et al., 2021). In this research study, Simulink software was used to build intelligent control systems using C language in programming, and AI was used to control the concentration of desflurane released. There were rules set by an anesthesiologist to make sure the concentration didn’t stray outside of the optimal range. The results of this study showed that the algorithm generated the desired value of anesthetic concentration. One limitation of this study was that there wasn’t a specific accuracy given on how often this value was generated, but the study did mention that the experiment should be implemented again using different anesthetic situations and gasses (Dominikowski et al., 2021).
During this experiment, the patient’s heart rate (HR) and mean arterial pressure (MAP) were constantly measured. The rules set by the anesthesiologist are decided based on what should be done in case of a change in HR or MAP. The AI system simply reads the HR and MAP and automatically sets the desflurane concentration based on what the anesthesiologist determined earlier (Dominikowski et al., 2021). If AI can be used to read vital signs for anesthetic concentrations, then it should be able to continuously monitor patients in the operating room and even in in-patient situations. A literature review was completed that showed AI and machine learning can be used to monitor and even predict vitals in a similar way (Shaik et al., 2023). This can be applied to remote patient monitoring (RPM), where the health of a patient can be analyzed and predicted even while they are at home. Patients with chronic illnesses or elderly patients at remote locations can still be monitored to make sure their condition doesn’t suddenly deteriorate. This application of AI will help to reduce the patient load in hospitals that still use manual monitoring of patient vitals, especially in rural locations (Shaik et al., 2023).
Unfortunately, there are also many challenges that come with RPM. Patient privacy and the uncertainty of how fast a patient’s condition can change can sometimes not be predicted. This means AI should still be supplemented with direct physician supervision rather than being fully autonomous, and certain rules and regulations need to be set on how much a patient’s information can be used for AI learning and monitoring (Shaik et al., 2023; Rudzicz et al., 2020).
Conclusion
Through this literature review, the exploration of the many benefits of using AI in surgery and patient care shines brightly. However, there are many challenges that also exist. Ethical issues such as privacy and autonomous control during surgery can be a tough hurdle to cross and require a slow and gradual change in the surgical setting and patient approval (Rudzicz et al., 2020). Many of these research studies had minimal live patient trials, which is very difficult to approve for testing. Another setback is that current AI algorithms aren’t as accurate as physicians and can contain algorithmic biases that affect how patients are diagnosed. Patients and physicians will need clarity on how AI makes decisions in a medical environment before it can be fully integrated into healthcare.
After researching the applications of AI in surgery, the ability to integrate this technology into healthcare is more efficient and effective than ever before. AI’s versatility extends to various areas within the healthcare industry, including the recognition of medical scans and their visual application over other surfaces. Augmented reality facilitates the alignment of scans over patients, streamlining surgical procedures and reducing time spent in the operating room. Moreover, AI can enhance laparoscopic surgery by analyzing videos and assisting in the identification and separation of nerves in the gastrointestinal system.
The benefits of AI extend beyond the operating room, with both image alignment and laparoscopic assistance contributing to shorter recovery times for patients. As surgeons complete tasks more rapidly, hospitals can accommodate more patients, addressing challenges such as overcrowded emergency rooms. Additionally, AI plays a crucial role in monitoring patient vitals and status, automating processes such as the adjustment of anesthetic gas levels based on heart rate and arterial pressure. This not only reduces the burden on hospitals but also enables remote patient monitoring and early detection of potential movement disorders or psychological disorders. These advancements represent a significant step forward in improving patient outcomes and streamlining healthcare processes. More research is required in this rapidly expansive industry, where the potential to improve patient care is endless (Mirnezami et al., 2018; Zhou et al., 2020). Once more research is completed, we will likely see the integration of AI to assist surgeons in operating and treating patients in a day-to-day setting.
Citations
Biebl, M., Alkatout, I., Biebl, M., & Alkatout, I. (2021). Recent Advances in Minimally Invasive Surgery. MDPI – Multidisciplinary Digital Publishing Institute.
Chu, J., Hsieh, C.-H., Shih, Y.-N., Wu, C.-C., Singaravelan, A., Hung, L.-P., & Hsu, J.-L. (2022). Operating Room Usage Time Estimation with Machine Learning Models. Healthcare (Basel), 10(8), 1518-. https://doi.org/10.3390/healthcare10081518
Crigger, E., & Khoury, C. (2019, February 1). Making policy on augmented intelligence in health care. Journal of Ethics | American Medical Association. https://journalofethics.ama-assn.org/article/making-policy-augmented-intelligence-health-care/2019-02
Dominikowski, B., & Gaszyński, T. (2021). Automatic control of desflurane concentration in surgical procedures using laparoscopic technique. Journal of Physics. Conference Series, 1782(1), 12003-. https://doi.org/10.1088/1742-6596/1782/1/012003
Kelly, C. J., Karthikesalingam, A., Suleyman, M., Corrado, G., & King, D. (2019). Key challenges for delivering clinical impact with artificial intelligence. BMC Medicine, 17(1), 195–199. https://doi.org/10.1186/s12916-019-1426-2
Kitaguchi, D., Takeshita, N., Matsuzaki, H., Oda, T., Watanabe, M., Mori, K., Kobayashi, E., & Ito, M. (2020). Automated laparoscopic colorectal surgery workflow recognition using artificial intelligence: Experimental research. International Journal of Surgery (London, England), 79, 88–94. https://doi.org/10.1016/j.ijsu.2020.05.015
Li, X., Tian, D., Li, W., Dong, B., Wang, H., Yuan, J., Li, B., Shi, L., Lin, X., Zhao, L., & Liu, S. (2021). Artificial intelligence-assisted reduction in patients’ waiting time for outpatient process: a retrospective cohort study. BMC Health Services Research, 21(1), 237–237. https://doi.org/10.1186/s12913-021-06248-z
Liao, R., Miao, S., de Tournemire, P., Grbic, S., Kamen, A., Mansi, T., & Comaniciu, D. (2016). An Artificial Agent for Robust Image Registration. arXiv.Org. https://doi.org/10.48550/arxiv.1611.10336
Mirnezami, R., & Ahmed, A. (2018). Surgery 3.0, artificial intelligence and the next‐generation surgeon. British Journal of Surgery, 105(5), 463–465. https://doi.org/10.1002/bjs.10860
Ryu, S., Goto, K., Kitagawa, T., Kobayashi, T., Shimada, J., Ito, R., & Nakabayashi, Y. (2023). Real-time Artificial Intelligence Navigation-Assisted Anatomical Recognition in Laparoscopic Colorectal Surgery. Journal of Gastrointestinal Surgery, 27(12), 3080–3082. https://doi.org/10.1007/s11605-023-05819-1
Ryu, S., Goto, K., Imaizumi, Y., & Nakabayashi, Y. (2024). Laparoscopic Colorectal Surgery with Anatomical Recognition with Artificial Intelligence Assistance for Nerves and Dissection Layers. Annals of Surgical Oncology, 31(3), 1690–1691. https://doi.org/10.1245/s10434-023-14633-7
Rudzicz, F., & Saqur, R. (2020). Ethics of Artificial Intelligence in Surgery. arXiv.Org. https://doi.org/10.48550/arxiv.2007.14302
Shaik, T., Tao, X., Higgins, N., Li, L., Gururajan, R., Zhou, X., & Acharya, U. R. (2023). Remote patient monitoring using artificial intelligence: Current state, applications, and challenges. Wiley Interdisciplinary Reviews. Data Mining and Knowledge Discovery, 13(2), e1485-n/a. https://doi.org/10.1002/widm.1485
Siemionow, K., Katchko, K., Lewicki, P., & Luciano, C. (2020). Augmented reality and artificial intelligence-assisted surgical navigation: Technique and cadaveric feasibility study. Journal of Craniovertebral Junction and Spine, 11(2), 81–85. https://doi.org/10.4103/jcvjs.JCVJS_48_20
Zhou, X.-Y., Guo, Y., Shen, M., & Yang, G.-Z. (2020). Application of artificial intelligence in surgery. Frontiers of Medicine, 14(4), 417–430. https://doi.org/10.1007/s11684-020-0770-0